Mathematical theorems are statements that can be proven to be true using logic and rules. They are the building blocks of mathematics, and they help us understand and solve problems in various domains, such as science, engineering, economics, and more. Some of the most famous examples of mathematical theorems are Pythagoras’ theorem, Fermat’s last theorem, and the Riemann hypothesis.
But how do mathematicians come up with these theorems in the first place? And Can AI Invent Mathematical Theorems? This is the main question we will explore in this article. We will look at how AI can assist mathematicians in discovering patterns, formulating conjectures, writing proofs, finding connections, and solving problems. We will also discuss some of the examples of mathematical breakthroughs achieved with the assistance of AI, as well as some of the challenges and implications of using AI to invent mathematical theorems.
So buckle up and get ready for a fascinating journey into the world of AI and mathematics. Let’s go! 🚀
Contents
- 1 Can AI Invent Mathematical Theorems?
- 2 How AI can prove mathematical theorems using automated methods
- 3 How AI can discover new mathematical theorems using machine learning
- 4 How AI can help mathematicians using chatbots and machine learning
- 5 How AI can create new mathematics using generative models
- 6 How AI can solve math problems using natural language processing
- 7 How AI can understand math using symbolic reasoning
- 8 How AI can learn math using reinforcement learning
- 9 Conclusion
Can AI Invent Mathematical Theorems?
Mathematics is a field that relies on human intuition, creativity and logic to discover patterns, formulate conjectures and prove theorems. However, in recent years, artificial intelligence (AI) has shown remarkable capabilities in assisting mathematicians with various aspects of their work. In this blog post, we will explore some of the ways that AI can invent mathematical theorems and how it can change the future of mathematics.
How AI can prove mathematical theorems using automated methods
One of the most basic tasks of mathematics is to prove that a conjecture is true for all possible cases. This requires rigorous logic and reasoning, which can be challenging for humans, especially for complex or abstract problems. AI can help by using automated methods to check the validity of proofs or to generate new proofs from scratch.
For example, a team from Google created an AI system that proved more than 1200 mathematical theorems. The system used a technique called reinforcement learning, which involves learning from trial and error. The system was given a set of axioms (basic rules) and a goal theorem to prove. It then tried to apply different logical steps until it either reached the goal or got stuck. The system received feedback based on whether its steps were valid or not, and learned to improve its strategy over time.
The system was able to prove theorems that were already known by mathematicians, but it could potentially tackle more difficult problems in the future. The system also generated proofs that were concise and elegant, which are desirable qualities for human mathematicians.
How AI can discover new mathematical theorems using machine learning
Another important aspect of mathematics is to find patterns and relations between mathematical objects, such as numbers, shapes or functions. These patterns can inspire new conjectures, which are statements that are suspected to be true but have not been proven yet. Conjectures can guide further research and lead to new discoveries.
AI can help by using machine learning techniques to analyze large amounts of data and extract useful information. Machine learning involves training a computer system to learn patterns from data without explicit instructions. The system can then use these patterns to make predictions or suggestions.
For example, a team from the University of Sydney used AI to suggest and help prove new theorems in representation and knot theory. Representation theory is a branch of mathematics that studies how abstract structures (such as groups or algebras) can be represented by concrete objects (such as matrices or functions). Knot theory is a branch of mathematics that studies properties of knots, which are loops that can be twisted or tangled in three-dimensional space.
The team used a type of machine learning called deep learning, which involves using artificial neural networks to learn complex features from data. The team trained their neural networks on data sets of representations and knots, and then used them to generate new examples of these objects. The team also used attribution techniques, which involve explaining how the neural networks make their decisions, to understand the underlying patterns and relations between the objects.
The team was able to discover a surprising connection between algebraic and geometric invariant knots, establishing a completely new theorem in the field. The team also proposed several new conjectures based on their observations.
How AI can help mathematicians using chatbots and machine learning
Mathematics is not only about finding proofs and conjectures, but also about communicating ideas, exploring possibilities and developing intuition. Mathematics is a human endeavor that requires collaboration and feedback from peers. AI can help by providing tools and platforms that facilitate mathematical reasoning and dialogue.
For example, a team from the University of California, Los Angeles explored how AI can change mathematics by using chatbots and machine learning to assist with mathematical reasoning. Chatbots are computer programs that can converse with humans using natural language. Machine learning is a type of AI that can learn from data and make predictions or suggestions.
The team developed several chatbots that could interact with mathematicians on various topics, such as number theory, geometry or logic. The chatbots could provide information, examples, hints or feedback based on the user’s input. The chatbots could also learn from previous conversations and improve their performance over time.
The team found that chatbots could be useful for teaching, learning and researching mathematics. Chatbots could help mathematicians to access relevant information
How AI can create new mathematics using generative models
AI is not only a tool or a partner for mathematicians, but also a potential source of inspiration and innovation. AI can challenge mathematicians to rethink their assumptions, methods and goals, and to explore new domains and possibilities. AI can also create new mathematics by generating novel mathematical objects or concepts that have not been seen before.
For example, a team from the University of Toronto used AI to create new mathematical structures called neural algebras. Neural algebras are a type of non-associative algebras that are defined by artificial neural networks. Non-associative algebras are algebras that do not satisfy the associative property, which means that the order of operations matters. For instance, in a non-associative algebra, (a+b)+c may not be equal to a+(b+c).
The team used a type of machine learning called generative adversarial networks (GANs), which involve two competing neural networks: a generator and a discriminator. The generator tries to produce realistic examples of neural algebras, while the discriminator tries to distinguish between real and fake examples. The generator and the discriminator learn from each other and improve their skills over time.
The team was able to generate new neural algebras that had interesting properties and structures. The team also studied the relations between neural algebras and other types of algebras, such as Lie algebras and Jordan algebras. The team suggested that neural algebras could have applications in physics, computer science and AI.
How AI can solve math problems using natural language processing
Mathematics is not only about abstract symbols and formulas, but also about natural language and communication. Mathematics involves expressing ideas, problems and solutions in words, as well as reading and understanding mathematical texts. AI can help by using natural language processing (NLP) techniques to process and generate mathematical language.
For example, a team from Google used AI to solve math problems written in natural language. The system used a technique called transformer-based neural network models, which involve using attention mechanisms to encode and decode sequences of words or symbols. The system was trained on a large corpus of math problems and solutions from various sources, such as textbooks, websites and exams.
The system was able to solve math problems ranging from arithmetic to calculus, as well as word problems that required reasoning and interpretation. The system could also generate step-by-step explanations for its solutions, as well as provide feedback and hints for incorrect or incomplete answers. The system could also handle different formats and styles of math problems, such as multiple choice or free response.
The system was designed to be a useful tool for students and teachers who want to practice or learn math. The system could also be extended to other domains or languages that involve mathematical reasoning.
How AI can understand math using symbolic reasoning
Mathematics is not only about computation and data, but also about symbols and logic. Mathematics involves manipulating symbols according to rules and principles, as well as proving or disproving statements using logical arguments. AI can help by using symbolic reasoning techniques to understand and manipulate mathematical symbols.
For example, a team from Stanford University used AI to understand math using symbolic reasoning. The system used a technique called symbolic program synthesis, which involves generating programs that can perform certain tasks or satisfy certain specifications. The system was given a set of mathematical symbols, such as variables, constants, operators and functions, as well as a set of constraints or goals, such as equations or inequalities.
The system was able to synthesize programs that could manipulate the symbols according to the constraints or goals. The system could also verify the correctness of its programs using formal methods. The system could also generalize its programs to handle different inputs or outputs.
The system was able to understand math at various levels of abstraction and complexity, from elementary algebra to differential equations. The system could also handle different types of math problems, such as simplification, integration or optimization. The system could also interact with humans using natural language or graphical interfaces.
The system was designed to be a powerful tool for mathematicians who want to explore or solve math problems using symbolic reasoning. The system could also be adapted to other domains or languages that involve symbolic manipulation.
How AI can learn math using reinforcement learning
Mathematics is not only about knowledge and skills, but also about learning and discovery. Mathematics involves acquiring new concepts, methods and techniques, as well as applying them to new situations or problems. AI can help by using reinforcement learning techniques to learn and discover math.
For example, a team from the University of Cambridge used AI to learn math using reinforcement learning. Reinforcement learning is a type of machine learning that involves learning from trial and error based on rewards or penalties. The system was given a set of math problems, such as equations or puzzles, as well as a set of actions, such as adding, subtracting or multiplying.
The system was able to learn how to solve the math problems by trying different actions and observing the outcomes. The system received rewards or penalties based on whether its actions led to correct or incorrect solutions. The system learned to improve its strategy over time by maximizing its rewards or minimizing its penalties.
The system was able to learn math at various levels of difficulty and diversity, from basic arithmetic to advanced algebra. The system could also handle different types of math problems, such as linear or nonlinear, deterministic or stochastic, discrete or continuous. The system could also adapt to different environments or goals, such as speed or accuracy.
The system was designed to be a flexible and robust tool for learning and discovering math. The system could also be applied to other domains or languages that involve problem-solving or decision-making.
Conclusion
AI is a powerful and versatile technology that can invent mathematical theorems in various ways. AI can prove, discover, help, create, solve and understand math using different techniques and approaches. AI can also change the future of mathematics by opening new horizons and possibilities.
AI is not a threat or a replacement for mathematicians, but a friend and a collaborator. AI can enhance and complement the human capabilities and creativity in mathematics. AI can also inspire and challenge mathematicians to explore new domains and problems.
Mathematics and AI are two fields that can benefit from each other and grow together. Mathematics can provide AI with foundations, methods and challenges. AI can provide mathematics with tools, platforms and insights. Mathematics and AI can work together to advance science and society.
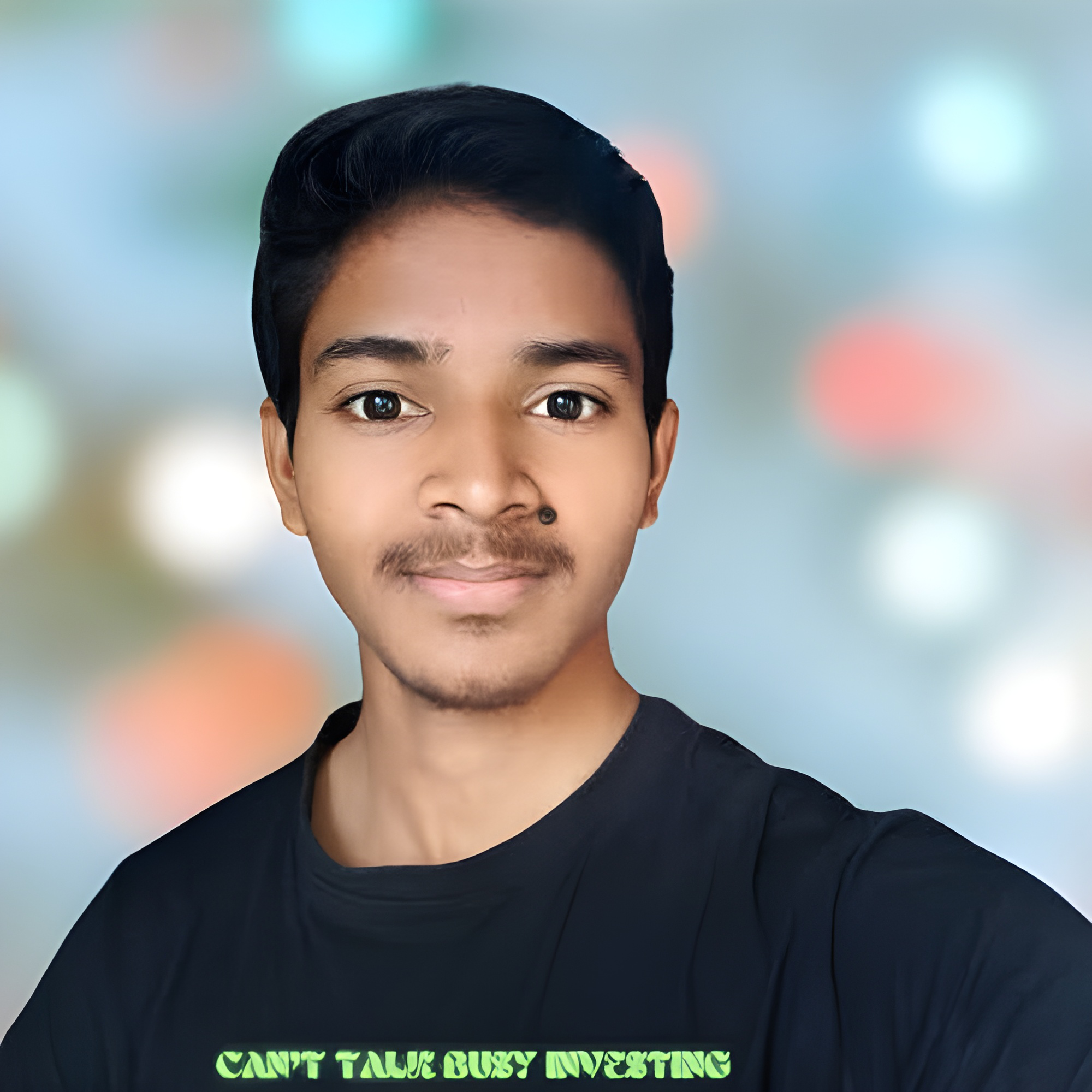
Hey there! I’m Kuldeep Kumar, and tech is my jam. From the mind-blowing world of AI to the thrilling battlegrounds of cybersecurity, I love exploring every corner of this amazing world. Gadgets? I geek out over them. Hidden software tricks? Bring ’em on! I explain it all in clear, bite-sized chunks, laced with a touch of humor to keep things sparky. So, join me on this tech adventure, and let’s demystify the wonders of technology, one blog post at a time.